AI develops human-like number sense – taking us a step closer to building machines with general intelligence

Gearstd/Shutterstock Adam Stanton, Keele University
Numbers figure pretty high up on the list of what a computer can do well. While humans often struggle to split a restaurant bill, a modern computer can make millions of calculations in a mere second. Humans, however, have an innate and intuitive number sense that helped us, among other things, to build computers in the first place.
Unlike a computer, a human knows when looking at four cats, four apples and the symbol 4 that they all have one thing in common – the abstract concept of “four” – without even having to count them. This illustrates the difference between the human mind and the machine, and helps explain why we are not even close to developing AIs with the broad intelligence that humans possess. But now a new study, published in Science Advances, reports that an AI has spontaneously developed a human-like number sense.
For a computer to count, we must clearly define what the thing is we want to count. Once we allocate a bit of memory to maintain the counter, we can set it to zero and then add an item each time we find something we want to record. This means that computers can count time (signals from an electronic clock), words (if stored in the computer’s memory) and even objects in a digital image.
This last task, however, is a bit challenging, as we have to tell the computer exactly what the objects look like before it can count them. But objects don’t always look the same – variation in lighting, position and pose have an impact, as well as any differences in construction between individual examples.
All the successful computational approaches to detecting objects in images work by building up a kind of statistical picture of an object from many individual examples – a type of learning. This allows the computer to recognise new versions of objects with some degree of confidence. The training involves offering examples that do, or do not, contain the object. The computer then makes a guess as to whether it does, and adjusts its statistical model according to the accuracy of the guess – as judged by a human supervising the learning.
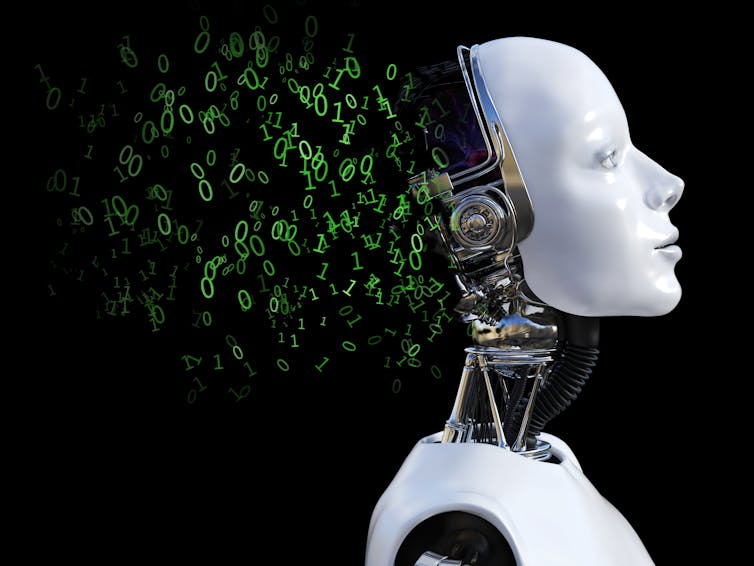
Modern AI systems automatically start to being able to detect objects when provided with millions of training images of any sort – just like humans do. These unsupervised learning systems gradually notice parts of the elements in the images that are often present at the same time, and build up layer upon layer of more complicated commonalities.
Take recognising apples as an example. As images containing all manner of shapes are presented to the system, it first begins to notice groups of pixels that make up horizontal and vertical lines, and left and right curves. They’re present in apples, faces, cats and cars, so the commonalities, or abstractions, are found early on. It eventually realises that certain curves and lines are often present together in apples – and develops a new, deeper level abstraction that represents a class of objects: apples, in this case.
Deep learning
This natural emergence of high-level abstractions is one of the most exciting results of the machine learning technique called deep neural networks, which in some sense work in a similar way to the human brain. The “depth” comes from the many layers in the network – as the information goes deeper into the network, the commonalities found become more abstract. In this way, networks are created with elements that are strongly active when the input is similar to what it has experienced before. The most abstract things appear at the deepest levels – these are cats, faces and apples rather than vertical lines or circles.
When an AI system can recognise apples, you can then use it to count how many there are. That’s great, yet it’s not quite how you or I would count apples. We have an extremely deep concept of “number” – how many of something there is. Rather than just being active when an object is present, parts of our brain activate depending on the amount of objects present. It means we can look at a bunch of apples and know that there are four without actually counting each one.
In fact, many animals can do this too. That’s because this sense of numerosity is a useful trait for survival and reproduction in a lot of different situations – take for instance judging the size of groups of rivals or prey.
Emergent properties
In the new study, a deep neural network that was trained for simple visual object detection spontaneously developed this kind of number sense. The researchers discovered that specific units within the network suddenly “tuned” to an abstract number – just like real neurons in the brain might respond. It realised that a picture of four apples is similar to a picture of four cats – because they have “four” in common.
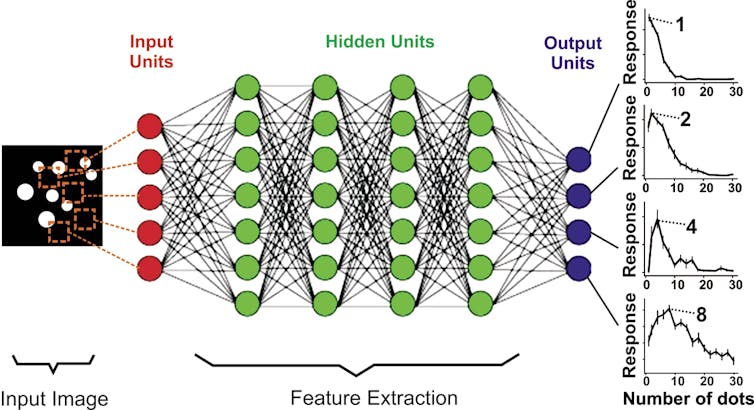
One really exciting thing about this research is that it shows that our current principles of learning are quite fundamental. Some of the most high-level aspects of thinking that people and animals demonstrate are related deeply to the structure of the world, and our visual experience of that.
It also hints that we might be on the right track to achieve a more comprehensive, human-level artificial intelligence. Applying this kind of learning to other tasks – perhaps applying it to signals that occur over a period of time rather than over pixels in an image – could yield machines with even more human-like qualities. Things we once thought fundamental to being human – musical rhythm for example, or even a sense of causality – are now being examined from this new perspective.
As we continue to discover more about building artificial learning techniques, and find new ways to understand the brains of living organisms, we unlock more of the mysteries of intelligent, adaptive behaviour.
There’s a long way to go, and many other dimensions that we need to explore, but it’s clear that the capability to look at the world and work out its structure from experience is a key part of what makes humans so adaptable. There’s no doubt it will be a necessary component of any AI system that has the potential to perform the variety and complexity of tasks that humans can.
Adam Stanton, Lecturer in Evolutionary Robotics and Artificial Life, Keele University
This article is republished from The Conversation under a Creative Commons license. Read the original article.
Most read
- Keele ranks among England’s Top 15 universities for combined research and teaching excellence
- Reunion for Keele's 'pioneer' graduates
- Keele Professor receives Lifetime Achievement Award for significant impact on healthcare research
- Keele researcher awarded Academy of Medical Sciences funding
- Previously unknown details of aphids in flight will contribute to improved crop security
Contact us
Andy Cain,
Media Relations Manager
+44 1782 733857
Abby Swift,
Senior Communications Officer
+44 1782 734925
Adam Blakeman,
Press Officer
+44 7775 033274
Strategic Communications and Brand news@keele.ac.uk.